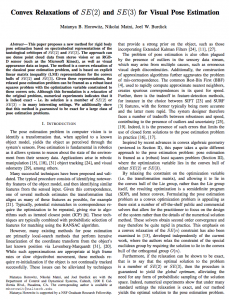
Convex Relaxations of SE(2) and SE(3) for Visual Pose Estimation
Today I read a published paper “Convex Relaxations of SE(2) and SE(3) for Visual Pose Estimation”
The abstract is:
This paper proposes a new method for rigid body pose estimation based on spectrahedral representations of the tautological orbitopes of $SE(2)$ and $SE(3)$. The approach can use dense point cloud data from stereo vision or an RGB-D sensor (such as the Microsoft Kinect), as well as visual appearance data. The method is a convex relaxation of the classical pose estimation problem, and is based on explicit linear matrix inequality (LMI) representations for the convex hulls of $SE(2)$ and $SE(3)$. Given these representations, the relaxed pose estimation problem can be framed as a robust least squares problem with the optimization variable constrained to these convex sets. Although this formulation is a relaxation of the original problem, numerical experiments indicate that it is indeed exact – i.e. its solution is a member of $SE(2)$ or $SE(3)$ – in many interesting settings. We additionally show that this method is guaranteed to be exact for a large class of pose estimation problems.