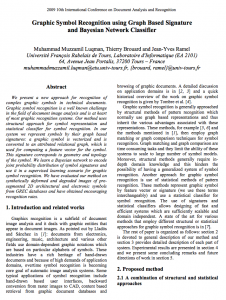
Graphic Symbol Recognition using Graph Based Signature and Bayesian Network Classifier
Today I read a published paper titled “Graphic Symbol Recognition using Graph Based Signature and Bayesian Network Classifier”
Interesting combination of a relational graph and a Bayesian network to recognise non-alphanumeric symbols in technical documents. As this is a reasonably hard problem to solve (or it was when I was doing that sort of thing about 20 years ago) it is encouraging to read new approaches (new for me anyway) to the problem. I wish the recognition rates (acknowledged in the paper) were a little higher.
The abstract:
We present a new approach for recognition of complex graphic symbols in technical documents. Graphic symbol recognition is a well known challenge in the field of document image analysis and is at heart of most graphic recognition systems. Our method uses structural approach for symbol representation and statistical classifier for symbol recognition. In our system we represent symbols by their graph based signatures: a graphic symbol is vectorized and is converted to an attributed relational graph, which is used for computing a feature vector for the symbol. This signature corresponds to geometry and topology of the symbol. We learn a Bayesian network to encode joint probability distribution of symbol signatures and use it in a supervised learning scenario for graphic symbol recognition. We have evaluated our method on synthetically deformed and degraded images of pre-segmented 2D architectural and electronic symbols from GREC databases and have obtained encouraging recognition rates.